Deciphering Groundwater-Surface water Interactions using Environmental Tracers in a Tropical Lake, Southwest India
1
Department of Environmental Sciences,
University of Kerala,
Thiruvananthapuram,
Kerala
India
2
Department of Water Engineering,
College of Agriculture,
Isfahan University of Technology,
Isfahan,
Iran
Corresponding author Email: jsabu@keralauniversity.ac.in
DOI: http://dx.doi.org/10.12944/CWE.19.3.5
Copy the following to cite this article:
Raghavan S. S, Joseph S, Eslamian S. Deciphering Groundwater-Surface water Interactions using Environmental Tracers in a Tropical Lake, Southwest India. Curr World Environ 2024;19(3). DOI:http://dx.doi.org/10.12944/CWE.19.3.5
Copy the following to cite this URL:
Raghavan S. S, Joseph S, Eslamian S. Deciphering Groundwater-Surface water Interactions using Environmental Tracers in a Tropical Lake, Southwest India. Curr World Environ 2024;19(3).
Download article (pdf)
Citation Manager
Publish History
Select type of program for download
Endnote EndNote format (Mac & Win) | |
Reference Manager Ris format (Win only) | |
Procite Ris format (Win only) | |
Medlars Format | |
RefWorks Format RefWorks format (Mac & Win) | |
BibTex Format BibTex format (Mac & Win) |
Article Publishing History
Received: | 2024-12-04 |
---|---|
Accepted: | 2024-12-29 |
Reviewed by: | ![]() ![]() |
Second Review by: |
![]() ![]() |
Final Approval by: | Dr. Gopal Krishan |
Introduction
Globally, lakes boast a colossal water storage capacity of ~42,320 cubic miles, sustaining around one billion people as their primary freshwater source.1 In the global warming scenario, a recent projection suggests that global lake evaporation will increase by approximately 4% per °K, marking a twofold rise compared to the ~2% per °K observed in global ocean evaporation.2 This phenomenon has contributed to the shrinkage and diminished water storage in numerous tropical lakes in recent years.3-6
As a consequence of high rates of evaporative losses without corresponding adjustments in water inflow, these lakes face a reduction in volumes and a downgrade in their essential functions.7,8 In alignment with UN Sustainable Development Goal 6, there is a global-level concerted effort underway to conserve these freshwater resources.9,10 However, achieving sustainable water management necessitates a profound grasp of various hydrological aspects. These include recharge-discharge processes, water residence and transit times, groundwater-surface water interactions etc.11-13
Recent literature has emphasized the significance of groundwater-surface water (GW-SW) interaction, particularly in a vast country like India grappling with a rapid decline in per capita water availability.14, 15 This study examines GW-SW interaction — a significant but frequently disregarded process that profoundly impacts the hydrological and chemical equilibrium of lakes. The GW-SW interaction is primarily controlled by various factors including topography, geology, climate, anthropogenic activities (e.g., landuse) etc.16-20 Therefore, comprehending the dynamic mechanism of this interaction is exceedingly complex in lake ecosystems. The process of groundwater inflow into lake, i.e., lacustrine groundwater discharge (LGD) is highly influenced by aquifer-lakebed heterogeneity, hydraulic gradients, and bedrock fractures etc.21-23
Some of the commonly employed methods for investigating groundwater discharge encompass EC, pH and temperature measurement, hydraulic analysis, electrical geophysical techniques etc.24, 25 While seepage meters offer precise point-scale information, their drawback lies in the potential lack of spatial representation. Numerous investigations have employed data-intensive numerical process-based approaches, proving less effective in regions lacking substantial data 26. In such data scarce regions, the imperative lies in effectively utilizing innovative techniques like isotope tracers for investigating GW-SW interaction processes.27
Stable isotopes, specifically s18O and s2H act as water tracers, providing vital information regarding recharge processes, evaporation, groundwater mixing etc.28 These isotopes serve as effective tools in understanding the hydrological cycle and play a vital role in assessing GW-SW interaction through end-element mixing models.29,30 The recent advent of machine learning (ML) techniques has proven beneficial across various facets of water resources management studies.31 Despite the potential effectiveness of integrating ML with stable isotope techniques in attaining high-resolution and reliable findings, such attempts remain limited in GW-SW connectivity studies.
Hydrochemical approaches are widely used to study connectivity between surface water and groundwater, assuming linear mixing and minimal chemical reactions between them.32, 33 Among hydrochemical tracers, chloride (Cl-) is particularly valuable as a conservative ion, unaffected by adsorption or degradation, making it ideal for tracing water movement through mass transport alone.34 By excluding anthropogenic inputs, chloride provides a conservative estimate for calculating groundwater discharge into the lake.35
Globally several studies on groundwater-surface water (GW-SW) interactions emphasize varied challenges and methodologies across different environmental contexts. Tigabu et al.16 used the SWAT-MODFLOW model to assess GW-SW dynamics in the Lake Tana Basin, Ethiopia, revealing flow pattern differences across catchments, attributed to variations in aquifer properties and morphology. Rodríguez-Rodríguez et al.17 examined GW-SW interactions in southern Spain’s Doñana National Park, finding that groundwater withdrawals, alongside reduced precipitation, led to pond degradation, outlining the importance of both natural and anthropogenic drivers in GW-SW studies.
In another study, the impact of extreme rainfall on GW-SW interactions and associated contamination risks were analyzed in Gaborone Dam catchment, Botswana18. Sun et al.21 studied lacustrine groundwater discharge (LGD) in East Dongting Lake, China, revealing that contrasting geological conditions resulted in differing LGD rates and nutrient loads21. These studies emphasize the necessity for context-specific approaches to understanding GW-SW interactions. The integration of stable isotopes and ML techniques offers potential for advancing this understanding, particularly in data-limited tropical lake ecosystems, where complex dynamics are often overlooked. This study attempts to address these gaps and contribute to sustainable water management in similar regions.
In the context of Vellayani Lake (VL) in Southwest India, this study aims to identify and delineate locations of GW-SW interactions in the lake, contributing nuanced within a tropical setting. As a preliminary study, it establishes a foundation for subsequent in-depth analyses, providing comprehensive assessments of the factors influencing the hydrological balance of VL. Additionally, amidst the recent drought episodes affecting Thiruvananthapuram city (SW India), where this lake is situated, the study holds relevance for sustainable water resources management crucial for the well-being of the thousands of people dependent on VL as the primary water source. While the study leverages isotopic and chloride mass balance methods to provide reliable understandings, it acknowledges the need for further integration of long-term datasets and advanced hydrogeological modeling to refine spatio-temporal assessments of GW-SW interactions. These considerations pave the way for future research to enhance the applicability and scalability of the findings in tropical lake ecosystems.
Materials and Methods
Study Area
The Vellayani Lake-VL (length= 3.15 km, Area= 2.25 km2), the second largest fresh water lake in Kerala, is located in the lowland physiographic province of Karamana River basin, Kerala, SW India. It is ~15 km from Thiruvananthapuram city (N. Lat. 08.45° and E. Long. 76.98°), Kerala. The region falls under a transitional climate between tropical monsoon (Am) and tropical savanna (Aw) classifications according to the Köppen climate classification system, exhibiting characteristics of both with significant rainfall during the monsoon season and a relatively distinct dry period. This elongated hammer-shaped lake body is aligned in the N-S direction and is roughly parallel to the modern shoreline, separated from the shore by a distance of ~ 4.0 km (Fig. 1). The total catchment area of lake is 38 km2 (Fig. 1).
The northwestern part of the lake is comparatively wider (width= 500 m) with a square shaped reservoir separated from the rest of the lake system by a breakable muddy barrack. From the reservoir part, the lake structurally takes a slight deviation towards southeastern direction and realigns to the N-S configuration with an elongated body shape in the tail end portion. The lake gets thinner towards the southernmost end with minimal inflows from the catchment terrain. The southern tail is divided by two bituminous roads, acting as physical barriers, dividing the lake body into three segments: Reservoir-Kakkamoola (RK; ~1.7 km), Kakkamoola-Vavvamoola (KV; ~1 km), and Vavvamoola-Panangode (VP; ~0.5 km) (Fig. 1).
Precambrian crystalline basement and Tertiary sediments constitute nearly 90% of the drainage basin of Vellayani Lake. Precambrian rocks are represented by khondalites and are confined to the eastern and southwestern part of the Vellayani basin. Charnockite, charnockitic gneiss and hypersthene-diopside-gneiss occur in the northeastern head region of the basin, Quaternary sediments constitute the NW side and Tertiary sediments constitute the southern side.36 Geomorphologically, Vellayani is a lowland lake (10-100 m) featuring ridge tops surrounded by moderate slopes. The eastern part is defined by dominant valleys, while the western portion is undulating with ridge tops on both sides.37 The northern boundary of the lake is dominated by irrigation.
![]() | Figure 1: Location map of Vellayani Lake along with sampling sites.
|
Sampling Scheme
In order to understand the lake water groundwater interaction, twelve lake water locations (V1-V12) were identified at an interval of ~200 m and two sets of lake water samples (one top layer i.e., L1-T to L12-T and one bottom layer of water i.e., L1-B to L12-B) were collected from each location. Bottom samples were collected using a bottom water sampler.
After a reconnaissance survey, a total of twelve wells were selected ~100-200 m away from the shore line of the lake within the catchment. Ground water samples (n= 12) were collected from shallow dug wells (<35 m) near shore of the elongated lake. Site selection was guided by pilot electrical conductivity surveys to assess the potential for groundwater discharge, complemented by hydrogeological mapping and proximity to groundwater abstraction sites. Accessible by land or boat, the number of sampling points provided comprehensive spatial representation across the northern, southern, eastern, and western regions of Vellayani Lake (VL), balancing coverage, logistical feasibility and encompassing variability in hydrological settings.
This approach provided a robust framework to investigate GW-SW dynamics while ensuring methodological rigor essential for hydrological assessments. The water samples (100 mL) were collected in high density polyethylene bottles without headspace. Stable isotope measurements in this study were executed using the Isotope Ratio Mass Spectrometer (Finnigan Delta PlusXP) at National Stable Isotope Facility at Centre for Water Resource Development and Management (CWRDM), India.
The isotopic composition of water is conventionally denoted in s notation, expressed in per mil (‰), relative to the international standard [s18O or sD = (Rsample/Rstandard - 1) × 1000], where R represents the abundance ratio of the heavy to light isotope [e.g., 18O/16O or 2H (or D)/1H] in the sample or standard. Stable water isotopes (s18O and s2H) are reported as per mill (‰) deviations from the 2H/1H and 18O/16O ratios based on Vienna Standard Mean Ocean Water (VSMOW). The overall external precision was determined to be ±0.5‰ for s18O and ±1.5‰ for sD. The portrait of the groundwater sampling sites is given in Supplementary Table S1. Further, the location map showing the lake water and ground water sampling sites are given in Fig. 1.
Chloride Mass Balance Approach
The chloride mass balance (CMB) method was employed to evaluate groundwater-surface water (GW-SW) interactions. This method was chosen due to its well-established reliability and simplicity in tracing groundwater fluxes using chloride concentrations, a conservative ion in natural waters that serves as a robust tracer for estimating water movement.
By complementing the stable isotope analysis, the chloride mass balance method provides an independent and corroborative estimation of groundwater interactions, thereby enhancing the reliability of the results. This synergy is particularly valuable for validating isotopic findings and ensuring robust interpretations. In data-scarce regions like the study area, the chloride mass balance method offers a practical and efficient alternative to more resource-intensive techniques.
To estimate changes in lake volume (VCL) due to various hydrological processes, a chloride mass balance model was employed.35 The equation utilized for this mass balance is:
where, CL, CG, CP and CR are the chloride concentrations of lake water, groundwater, precipitation and river water, respectively35
Gi, Pi, Ri, Ro and Ho represent the respective volumes (m3) for groundwater discharge, precipitation, river inflow, evaporation and consumption.35
Top of Form
2.4 Methodological Framework for Clustering and Predictive Modeling
The investigation of groundwater-surface water interaction patterns in the present study entailed the application of the k-means clustering algorithm, meticulously implemented using the R language with ©Rstudio (Version 4.3.1). Visualization was done using clusplot package of ©Rstudio.38 This methodological approach was meticulously selected for its efficacy.
K-means clustering, revered for its capacity to partition multidimensional datasets into distinct clusters based on similarities in isotopic compositions, was deemed the optimal analytical framework for discerning GW-SW interactions. The initial dataset underwent rigorous preprocessing to ensure data integrity and consistency, prerequisites for robust computational analysis.
The k-means clustering algorithm was then applied independently to both the top and bottom layers of the lake. This unsupervised learning methodology, predicated on the minimization of within-cluster sum of squares, served as the cornerstone for optimizing the homogeneity of resultant clusters. The parsimonious inclusion of pertinent isotopic variables emphasized the criticality of capturing hydrological intricacies intrinsic to the lake’s ecosystem. The algorithm’s initialization with a seed for reproducibility, coupled with iterative convergence, ensured the attainment of stable and convergent cluster assignments. The visualization of resultant clusters through scatter plots facilitated an intuitive apprehension of spatial isotopic patterns, offering a cogent framework.
The number of clusters was optimized based on the elbow method to ensure meaningful groupings, while preprocessing steps, such as standardization, ensured comparability across variables. The k-means clustering methodology assumed that isotopic compositions (s¹8O and sD) accurately represented the hydrological processes governing groundwater-surface water interactions. It presumed that clusters were well-separated in multidimensional isotopic space and exhibited inherent homogeneity, allowing distinct hydrological signatures to emerge. The spherical distribution of data points within clusters and the absence of significant overlap were foundational to achieving robust cluster assignments. Furthermore, the algorithm relied on the isotopic dataset’s ability to capture spatial and vertical variations across the lake’s ecosystem, ensuring that clustering outcomes aligned with hydrodynamic processes. These assumptions underpinned the reliability of k-means in partitioning the isotope dataset and elucidating groundwater-lake water interactions effectively.
Subsequently, a random forest (RF) classification model was employed to categorize sites into lacustrine groundwater discharge (LGD) and non-LGD groups. The RF algorithm, a powerful ensemble learning technique, operates by constructing multiple decision trees during training and aggregating their predictions to improve classification performance. The objective function of the RF method aims to minimize classification errors by selecting optimal split points at each node of the decision trees, based on measures like Gini impurity or information gain, and combining predictions from multiple trees to enhance robustness and accuracy. Random Forest (RF) classification was applied with 500 decision trees and model accuracy was assessed through a confusion matrix. To assess the model’s performance, a cross-validation approach was implemented. The dataset was divided into training and testing sets, enabling iterative training and validation to evaluate the model’s accuracy in predicting LGD and non-LGD classifications.
To validate the chloride mass balance model, predicted values of water fluxes (such as Lacustrine Groundwater Discharge and lake-to-groundwater recharge) were compared with observed values at 12 stations. The model’s performance was assessed using Mean Absolute Error (MAE), Root Mean Square Error (RMSE) and Coefficient of Determination (R2).
This study applied a predictive modeling framework combined with One-at-a-Time (OAT) Sensitivity Analysis to examine the influence of key hydrological parameters —groundwater discharge, lake-to-groundwater recharge, precipitation, evaporation, and pumping—on the lake volume. The model aimed to predict changes in lake volume under variable conditions of groundwater discharge (LGD), precipitation, evaporation, and pumping. Each parameter was altered by ±20% independently39, with other factors held constant, allowing for a focused examination of the system's responsiveness to individual variations.
The lake volume predictions were conducted using baseline values for each parameter, representing observed or measured rates, and then recalculated with the adjusted values for each input. The OAT approach allowed for isolating the impact of each parameter, thereby quantifying its influence on the hydrological balance.
The percentage change in the predicted lake volume was then calculated to quantify the system’s responsiveness to changes in these inputs. The following equation was used to determine the percentage variation in lake volume:
where Vadjusted represents the predicted lake volume under the adjusted parameter, and Vbaseline is the lake volume under baseline conditions.
This approach provided insights into the relative sensitivity of the lake’s hydrological balance to each parameter, helping prioritize factors critical for effective lake management.
To enable normalized comparison, we then calculated the sensitivity indicator/sensitivity index (SI) for each parameter using:
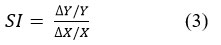
where Y represents the lake volume under baseline conditions, X represents the baseline value of each input parameter, Y is the observed change in lake volume from the baseline, and X corresponds to the ±20% adjustment of the parameter’s baseline value. The SI thus provides a standardized measure of sensitivity, allowing for identification of the parameters with the greatest relative impact on lake volume.
This methodology provides a thorough framework for understanding the dynamics of lake water management in a tropical context, integrating hydrological modeling, sensitivity analysis, and sustainability principles to inform effective resource allocation strategies.
Results and Discussion
The results of the stable water isotope (s18O and sD) analyses are presented in Table 1.
Table 1: Results of s18O (‰) and sD (‰) for Lake water samples and corresponding Groundwater samples
Sl.No. | Lake location | Lake water sample ID (Top layer) | s18O (‰) | sD (‰) | Lake water sample ID (Bottom layer) | s18O (‰) | sD (‰) | Corresponding Groundwater Sample ID | s18O (‰) | sD (‰) |
1 | V1 | L1-T | -0.33 | -6.35 | L1- B | -0.35 | -5.74 | G10 | -0.32 | -3.90 |
2 | V2 | L2-T | -0.10 | -2.09 | L2 B | -0.12 | -4.06 | G11 | -4.20 | -22.09 |
3 | V3 | L3-T | -1.11 | -5.66 | L3-B | -1.85 | -5.82 | G12 | -1.02 | -5.60 |
4 | V4 | L4-T | 0.00 | -4.13 | L4-B | -0.13 | -4.35 | G9 | -4.57 | -25.56 |
5 | V5 | L5-T | -0.12 | -4.36 | L5-B | -0.01 | -3.38 | G8 | -4.55 | -25.33 |
6 | V6 | L6-T | -0.01 | -4.05 | L6-B | -0.11 | -3.81 | G7 | -4.14 | -21.43 |
7 | V7 | L7-T | -0.09 | -4.25 | L7-B | -0.05 | -4.49 | G6 | -4.33 | -22.25 |
8 | V8 | L8-T | -0.25 | -3.94 | L8-B | -0.36 | -5.30 | G5 | -4.52 | -23.07 |
9 | V9 | L9-T | -0.42 | -4.43 | L9-B | -0.51 | -5.53 | G4 | -4.07 | -19.55 |
10 | V10 | L10-T | 0.06 | -2.80 | L10-B | 0.14 | -2.93 | G3 | -4.17 | -20.43 |
11 | V11 | L11-T | -0.17 | -3.24 | L11-B | -0.09 | -2.83 | G1 | -4.49 | -23.50 |
12 | V12 | L12-T | -0.77 | -6.25 | L12-B | -0.54 | -5.46 | G2 | -4.15 | -19.44 |
Observed patterns of stable water isotopes
Groundwater s18O values ranged from -4.57 to -0.32‰ (mean = -3.59‰) and sD values spanned from -25.56 to -3.9‰ (mean = -18.92‰). For lake water, the s18O values varied from -1.11 to 0.06‰ (mean = -0.24‰) for the top layer and -1.85 to 0.14‰ (mean = -0.33‰) for the bottom layer; whereas sD values fluctuated between -6.35 and -2.09‰ (mean = -4.21‰) for top layer and-5.82 to -2.93‰ (mean = -4.54‰) for bottom layer. A visual representation of the s18O and sD data for both surface water and groundwater is provided in the s18O - sD bivariate plot (Fig. 2).
An important observation related to this aspect is that majority of the groundwater samples (n=10) closely align with the Local Meteoric Water Line (LMWL), suggesting a predominant influence of precipitation on their isotopic composition. This is evidenced by the depleted s18O (-4.57 to -4.07‰; mean = -4.4‰) and sD (-25.56 to -19.44‰; mean = -22.26‰) values observed in these groundwater samples. This alignment indicates that the groundwater in the study area is primarily derived from meteoric water sources, emphasizing the importance of precipitation in replenishing the groundwater.40 The proximity of these samples to the LMWL also implies a consistent and uniform isotopic signature across the groundwater locations, reinforcing the notion of a shared hydrological origin influenced by meteoric input.
In the ensemble of 12 groundwater locations examined, two locations i.e., G10 and G12 positioned within the northern sector of the lake, manifested distinct isotope signatures that diverged significantly from the remaining groundwater samples. A visual examination of the s18O-sD bivariate plot (Fig. 2) reveals a discernible departure of these samples from both the Global Meteoric Water Line (GMWL) and Local Meteoric Water Line (LMWL). Unlike the majority of groundwater samples that display indications of recharge from precipitation, G10 and G12 exhibit an enrichment trend. Notably, despite their proximity to lake water samples, these groundwater samples appear depleted when compared to lake water samples adjacent to their respective locations i.e., L1 and L3 (Table 1, Fig.2). This observation supports the inference that these groundwater samples are not recharged by lake water. Further investigation, perhaps through detailed hydrogeological mapping and tracer studies would be necessary to fully understand the mechanisms behind these observations.
This intriguing observation is elucidated by the irrigational activities along the northern boundary of the lake, where G10 and G12 are situated. The distinctive isotopic signature of these groundwater samples is attributed to their recharge by irrigation return flow.41,42 Irrigation return flow water typically carries an enriched isotopic composition, providing a plausible explanation for the observed enrichment in G10 and G12 compared to the rest of the groundwater samples. This shows the influence of anthropogenic activities, specifically irrigation practices, on the isotopic dynamics within the groundwater-lake water system in the northern region of the study area. While specific studies directly supporting this correlation may be limited in our study area, our findings serve as compelling evidence, prompting further inquiry into this interrelationship between human interventions and hydrological processes. This recognition of the current research gap emphasizes the urgent need for future investigations to validate and expand upon our findings, thereby advancing our understanding of water resource management in anthropogenically influenced environments.
In contrast, the isotopic composition of lake water samples suggests a different process influencing their composition. All the lake water samples are enriched (lake water top layer s18O = -1.11 to 0.06‰, sD = -6.35 to -2.09‰; bottom layer water s18O = -1.85 to 0.14‰, sD = -5.82 to -2.93‰) than groundwater samples. The lake water samples plot distantly from both the LMWL and the global meteoric water line (GMWL), indicating a significant influence of evaporation processes on the lake water isotopic signature. The distinctive enriched stable water isotope signature of lake water primarily through evaporation signifies a complex relationship of internal processes.43 Evaporation-driven recycling within the system contributes to the observed deviation from typical isotopic patterns. Furthermore, the isotopic data from both the surface layer and bottom layer of lake water samples show a close clustering, suggesting a well-mixed condition within the lake. This uniformity can be attributed to the shallow depth (~2.0 meters) of the lake and the persistent windy conditions experienced for most parts of the days, facilitating efficient mixing throughout the water column.
The divergence in isotopic composition between lake water and groundwater samples demonstrates the intricate hydrological dynamics in the study area. While groundwater appears to be predominantly sourced from meteoric input, lake water undergoes a distinct evaporative process, contributing to its unique isotopic fingerprint (Fig. 2). This nuanced interpretation not only enhances our understanding of local water sources but also provides information on the role of precipitation and evaporation in controlling the isotopic composition of surface and groundwater in the region.
In certain instances, the observed isotopic enrichment hints at a potential interaction, suggesting the possibility of the upwelling of older, mineralized groundwater with longer residence times which may contribute to the distinctive isotopic composition observed in the lake.44,45 Efficient groundwater tracers like radon (222Rn) and radium (226Ra) could further enhance the understanding of groundwater interactions and isotopic enrichment within this nearly closed lake.
![]() | Figure 2: ?18O- ?D bivariate plot showing groundwater and lake water samples, VL.
|
Deuterium excess factor
The deuterium excess (d-excess) defined by Dansgaard46 is as follows: d = s2H – 8s18O. The d-excess values within the groundwater samples ranged from -1.34 to a 13.76‰, with a mean value of 10.34‰. The groundwater samples displaying the highest s18O and sD values, accompanied by lowest d-excess values suggest substantial evaporation before recharge. Notably, the minimum value is associated with G10 (d-excess = -1.34‰) and G12 (d-excess = 2.56‰), located in close proximity to irrigational site, indicating a potential evaporation-domination influence. This could be attributed to groundwater recharge from irrigation return flow at these sites which corroborates with the findings of s18O and sD data.
Conversely, the remaining groundwater samples, characterized by low s18O values and d-excess values exceeding 10‰, indicate rapid infiltration of water with minimal evaporation or direct recharge from precipitation. The maximum value (d-excess = 13.76‰) is observed at G2 which is located at SW shore of the lake. These contrasting isotopic signatures emphasize the influence of evaporation and precipitation dynamics on groundwater composition in the study area.
In the upper layer lake water samples (L1-T to L12-T), the d-excess values ranged from -4.13‰ to 3.22‰ (mean = -2.09‰). Negative d-excess values are typically associated with processes such as evaporation, where lighter isotopes preferentially evaporate, leading to a more enriched composition. The low d-excess values (< 5‰) in majority of the upper layer lake water are indicative of a longer residence time, allowing for increased evaporative effects47-49. The d-excess values in the bottom layer lake water (L1-B to L12B) were found to be in the range between -4.09‰ and 8.98‰ (mean = -1.82‰). The higher positive value (d-excess = 8.98‰) at L3-B in the bottom layer may indicate less evaporation.50
The deuterium excess (d-excess) value provides important information on non-equilibrium isotopic effects within the hydrological system. By capturing the disparity between s18O and sD, d-excess serves as a key indicator of the non-equilibrium degree during processes such as evaporation and condensation.51 The concept of d-excess hinges on the differential fractionation of 18O and D, offering an in-depth understanding of the hydrological dynamics.52
Climate conditions exert a substantial influence on d-excess values, where parameters such as temperature, relative humidity, and wind velocity modulate moisture source characteristics via water molecule diffusion processes.11,53 Differing from precipitation, the d-excess of groundwater is influenced by additional factors such as mixing with other water sources, evaporation during infiltration, residence time etc. owing to the distinctive hydrological conditions of the local environment.49, 54, 55 Previous research has indicated that integrating the vertical gradient of both s18O and d-excess could offer a promising method for refining the delineation of recharge areas within aquifer systems, particularly in mountainous environments.56
Groundwater-Surface Water Interactions
The results of the isotope analyses also provide valuable information on interaction between the lake water and groundwater. The isotopic composition indicates the occurrence of lacustrine groundwater discharge from G10 and G12 into the lake, specifically at sites V1 (L1-B) as well as V3 (L3-T) of the northern part of the lake (Fig. 2).
The groundwater sites G10 and G12 are characterized by laterite lithology (Supplementary Table 1) and down slope direction indicating lacustrine groundwater discharge (LGD). Laterite is a highly porous rock formation, often formed in tropical climates through weathering processes.57 Its characteristic high permeability allows water to flow more easily through the subsurface. In contrast, the majority of other sites in the study area have laterite combined with clay. Clay is known for its low permeability and can act as a barrier, hindering the movement of water through the subsurface.58 The presence of laterite lithology at sites G-10 and G-12 without the accompanying clay, in contrast to the majority of other sites in the study area (Supplementary Table S1), suggests a distinct hydrogeological influence on the interconnectivity between groundwater and lake water at these locations.
In the case of G-10, one plausible explanation is the existence of subsurface pathways or geological features that facilitate a direct exchange of water between G10 (s18O = -0.32‰; sD = -3.9‰) and L1-B (s18O = -0.35‰; sD = -5.74‰). Local hydrogeological conditions, such as permeable layers or preferential flow paths, topography etc. could be linked to the GW-SW interaction at this site. Generally, groundwater discharges into the lake through sediments, imparting its signature to the lake’s bottom water layer. Consequently, regions with pronounced lacustrine groundwater discharge (LGD) exhibit more depleted s18O and s2H values in the water layer near lakebed. Furthermore, the observed coarser sediment particles within the lakebed in the NE and NW region manifest the potential enhancement of lakebed permeability, fostering conditions conducive to lateral groundwater discharge (LGD). A permeable lakebed could suggest an intensified LGD.59
The connectivity of groundwater sample G12 (s18O =-1.02‰; sD = -5.6‰) to lake water sample L3-T (s18O = -1.11‰; sD = -5.66‰) was found to be relatively significant compared to L3-B. This isotope signature may be indicative of preferential interactions between G12 and the top layer of lake water (L3-T). Several hydrogeological factors could contribute to this observed isotopic relationship. There might be a permeable layer in close proximity to G12 that facilitates a more direct connection to the top layer of the lake.
The hydrogeological pathways between G12 and L3-T could be more direct, allowing for a quicker exchange of water. This might result in a more immediate influence on the groundwater from the top layer of the lake compared to the bottom layer. Local flow dynamics, influenced by geological structures or preferential flow paths, may contribute to a stronger hydraulic connection between G12 and L3-T.60 The presence of localized subsurface features, such as faults or geological heterogeneities, could create conditions that enhance the connectivity between G12 and the top layer of the lake. These features may act as conduits for groundwater movement, influencing the interaction dynamics.61,62 Further research is essential to comprehensively understand the underlying processes associated with the observed aspect. Based on the isotope data, a conceptual framework based on process-based principles was developed to visually represent and underpin the diverse hydrological processes within the northern part of VL (Fig. 3). This “hotspot” region holds significance, necessitating a targeted focus on groundwater augmentation measures for sustainable lake management.
![]() | Figure 3: Conceptual diagram of various hydrological processes controlling northern “hotspot” part of VL; (1) Groundwater recharge from irrigation return flow, (2) Lacustrine Groundwater Discharge, (3) Evaporation
|
Aquatic vegetation, particularly macrophytes also plays a pivotal role in lacustrine groundwater discharge (LGD) by generating preferential flow paths through root-induced cracks.21, 63 This mechanism facilitates lateral groundwater movement. However, the effectiveness of this process depends on vegetation density. Abundant macrophyte vegetation in the northern part of the lake suggests a correlation with enhanced lakebed permeability. While a limited number of studies illustrate an inverse correlation between aquatic vegetation and groundwater discharge, exemplified by increased seepage following the removal of vegetation cover64, a more prevalent observation is the positive association of these plants with groundwater seepage.
Numerous studies have consistently recognized the presence and distribution of aquatic plants, particularly in nearshore areas with floating-leaf and emergent vegetation, as valuable markers for identifying groundwater discharge locations within lacustrine environments.65-67 Nonetheless, a more detailed investigation in regions with partial vegetation coverage especially in the central and certain southern parts of the lake is necessary to understand the influence of this factor.
Climate variables, particularly precipitation and evaporation alter LGD volume and dynamics in shallow lakes.22 In tropical climates like the study area, persistent evaporation widens the gap between groundwater and lake water levels, intensifying LGD. In both LGD and non-LGD environments, the hydrological response to local rainwater manifests distinctive features.68
In LGD locales, local rainfall promptly elevates surface water levels, yet this precipitation undergoes processes of infiltration and lateral subsurface flow before ultimately contributing to groundwater discharge into the lake.69 As groundwater primarily discharges into the shallow areas of a lake, the sediments in the nearshore region serve as critical locations for various biogeochemical processes. These processes have the potential to transform, sequester, or even eliminate groundwater pollutants before they reach the lake water.70
On the other hand, non-lacustrine groundwater discharge (non-LGD) locations are distinguished by minimal topographic relief and low permeability of lakebed sediments compared to the northern sector of Vellayani Lake. This results in a significantly prolonged residence time, impeding the inflow of groundwater into the lake.59 Therefore, the protracted duration of groundwater residence distinctly decouples precipitation events from the dynamics of lacustrine groundwater discharge in non-LGD regions. Anthropogenic activities other than irrigational activities also influence LGD. For instance, intense groundwater extraction and depression cone formation reduces LGD intensity.30 These factors, particularly the impact of intense nearshore groundwater pumping warrant comprehensive investigation in future research.
Clustering
Prior to clustering, a pivotal phase involved the meticulous identification of groundwater discharge zones. This entailed a meticulous examination of isotopic data points, scrutinizing instances where groundwater s18O exhibited discernibly depleted values relative to the corresponding lake water layer. This discernment was instrumental in refining subsequent cluster assignments and enriching the interpretative depth of GW-SW interactions in VL. The clusplot of k-means clustering results indicated that the identified components effectively account for 85.77% of the variability observed among the data points, suggesting a substantial level of explanatory power in the clustering analysis.
The application of k-means clustering revealed two clusters in the study area. These clusters were distinctive, each bearing unique isotopic signatures in the study area. This categorization provided a comprehensive delineation of isotopic heterogeneity within non-LGD sites and LGD sites.
LGD Sites (Cluster 1)
Spatially, this cluster confirmed locations significantly influenced by underlying groundwater sources. A smaller subset of samples, approximately two data points, was identified as LGD sites. Cluster 1 exhibited groundwater samples with s18O values ranging from -1.02 to -0.32‰ and sD values from -5.60 to -3.90‰. The lake water s18O values in this cluster ranged from -1.85 to -0.33‰, whereas sD values from -6.35 to -5.66‰. This cluster clearly represented locations characterized by groundwater discharge into the lake (Fig. 4).
Non-LGD Sites (Cluster 2)
In this category, 10 data points formed a cohesive cluster identified as non-LGD sites (Fig. 4). The distinct circular boundary surrounding this cluster indicated a spatially segregated group less influenced by direct groundwater inputs. The s18O values of groundwater samples identified in cluster 2 were found to be varying from -4.57 to -4.07‰ and the sD values spanned from -25.56 to -19.55‰. In this cluster, the lake water s18O values were in the range -0.77 to 0.14‰, while the s18D values fluctuated between -6.25 to -2.09‰.
The RF classification model, trained on the clustered dataset, demonstrated exceptional performance during cross-validation. The resampling results across various mtry values, namely 2, 3, and 4, consistently revealed a good accuracy (0.9) and kappa (0.8) values during cross-validation. This exceptional performance led to the selection of the optimal model based on accuracy, with the final chosen configuration having mtry = 3.
![]() | Figure 4: K-means cluster plot of LGD and non-LGD sites, Vellayani Lake.
|
With an optimized parameter setting (mtry = 3), the model achieved an accuracy of 90% in predicting LGD and non-LGD classifications. The selection of this specific value was driven by the aim to maximize accuracy while maintaining a balance in variable selection. This method highlighted the significance of including additional variables in the random forest model, ultimately improving its predictive performance. The Kappa value of 0.8 further reinforces the model’s reliability, indicating a strong agreement beyond chance.
This remarkable performance illustrates the robustness of the random forest (RF) classification model in distinguishing between different categories. In the context of Lacustrine Groundwater Discharge (LGD), the model’s ability to consistently achieve perfection in accuracy and kappa values across different feature subsets is particularly noteworthy.
The uniform excellence in model performance indicates a strong association between stable isotope features, specifically s18O and sD, and the identification of LGD sites. The isotopic signatures within the identified clusters align with expectations for LGD, further supporting the model’s capability to discern specific variations.
The exceptional accuracy and kappa values across various tuning parameters establish the model’s effectiveness as a tool for hydrogeologists studying LGD processes. By providing a precise and consistent classification, the integration of Random Forest with clustering technique points out the potential for enhanced classification outcomes when utilizing this combined approach. The findings contribute valuable insights to the field of hydrogeological studies, offering a reliable approach to identify and characterize LGD sites with implications for environmental and water resource management.
Chloride Mass Balance
The calculated Lacustrine Groundwater Discharge (LGD) rates at V1 and V3 were 2.14×106 m3/yr and 3.82×106 m3/yr respectively. These LGD volumes indicate substantial groundwater inflow into the lake, supporting its overall water balance. These discharge volumes demonstrate the lake’s reliance on LGD in maintaining its water balance, particularly in the absence of riverine inputs. In this study, these “hotspots” are identified as critical zones where substantial groundwater discharge significantly impacts the lake ecosystem and are integral to understanding the influence of both anthropogenic factors and climatic conditions. By characterizing these regions, the findings provide essential information for enhancing the precision of future monitoring efforts.
On the other hand, the chloride mass balance analysis also revealed significant lake-to-groundwater recharge rates at stations V10 and V12, with estimated values of 7.01×106 m3/yr and 6.3×106 m3/yr, respectively. This suggests that these zones function as important conduits for groundwater recharge from lake to the surrounding aquifers.
This is particularly evident from their relatively lower chloride concentrations in groundwater compared to lake water (e.g., 25 mg/L in groundwater and 38 mg/L in lake water for V10), implying the role of lake water recharge into the aquifer (Table S2). The mass balance analysis for these stations shows that the lake serves not only as a discharge zone but also as a source of recharge for surrounding groundwater systems.
This trend of recharge provides quantitative evidence of the lake’s role in replenishing the surrounding aquifer towards the SW part. The comparison between stations shows spatial variability in both LGD and recharge, which likely reflects local heterogeneity in subsurface permeability, hydraulic gradients, and groundwater flow paths. This differentiation has significant implications for water resources management, particularly in identifying regions of groundwater extraction or conservation, depending on the discharge and recharge dynamics.
The validation of the chloride mass balance model revealed robust performance metrics. The Mean Absolute Error (MAE) was calculated at 0.28×106 m3/yr, indicating a strong alignment between the predicted and observed values. The Root Mean Square Error (RMSE) was determined to be 0.35×106 m3/yr, further demonstrating the model’s accuracy in estimating chloride concentrations. Additionally, the coefficient of determination (R2) was found to be 0.91, suggesting that 91% of the variability in the observed chloride concentrations can be explained by the model. These results confirm the reliability of the chloride mass balance model in estimating groundwater discharge and recharge within the study area.
Predictive Modeling of Parameter Influence
The results of the predictive modeling combined with One-at-a-Time (OAT) Sensitivity Analysis revealed distinct sensitivities of lake volume to variations in key hydrological parameters, allowing for a better understanding of how variations in these parameters affect the overall hydrological dynamics of the system. A ±20% change in groundwater discharge resulted in an approximate variation of 10.64% in lake volume, with a sensitivity index (SI) of 0.5320, implying LGD as “High” sensitivity parameter (Table 2). This reflects the significant role that groundwater discharge plays in maintaining lake water levels.
Additionally, a ±20% change in precipitation resulted in an approximate variation of 16.69% in lake volume. This affirms the sensitivity of lake volume to changes in precipitation inputs, emphasizing its critical role in the water balance of the lake system. This substantial variation is accompanied by a sensitivity index (SI) of 0.8345, classifying precipitation under “High” sensitivity (Table 2).
Table 2: Results of Predictive Model based on OAT Sensitivity Analysis
Parameter | Percentage Variation (±20%) | SI | Classification |
Precipitation | 16.69% | 0.8345 | High |
LGD | 10.64% | 0.5320 | High |
Evaporation | 7.48% | 0.3740 | High |
Pumping | 1.30% | 0.0650 | Medium |
Similarly, a ±20% change in evaporation was found to result in a variation of 7.48% in lake volume with an SI of 0.3740 (“High” sensitivity parameter), indicating that increased evaporation rates can significantly deplete water levels, thereby impacting overall lake health (Table 2). Conversely, reducing evaporation could aid in retaining more water, thus benefiting the lake ecosystem.
Finally, with a ±20% change resulting in a variation of 1.3% in lake volume, pumping emerged as the least sensitive parameter, exhibiting an SI of 0.0650 (“Medium” sensitivity parameter). While this effect may appear modest compared to other parameters, it shows the cumulative impact of unscientific water extraction practices on the lake’s hydrological budget.
Overall, the findings from this predictive modeling framework, combined with the OAT sensitivity analysis emphasize the critical nature of groundwater discharge and precipitation as the most sensitive parameters affecting lake volume. Effective management of these factors is essential for maintaining the ecological balance and hydrological integrity of the lake system. These results also emphasize the need for integrated water resource management strategies to sustain lake volume effectively. Understanding the sensitivity of these variables is crucial for informed decision-making regarding water allocation and conservation measures, particularly in the face of climate variability and increasing water demand.
Given these considerations, the potential future effects of climate change on VL can be anticipated. Global projections indicate shifts in precipitation patterns and an increase in climate extremes, particularly drought conditions, which are likely to influence the lake water levels significantly.71, 72
In line with the investigation of Goharian et al.73, who assessed the impact of various factors on the vulnerability of water supply systems in response to climate variability, this study elucidates the critical role of specific parameters in influencing the water dynamics of VL. More recently, the one-factor-at-a-time (OAT) sensitivity analysis conducted by Wang et al.74 demonstrated how variations in hydraulic conductivity, rainfall infiltration coefficients, and surface water levels significantly affect groundwater storage, thereby enhancing the understanding of groundwater-surface water interactions, which are pivotal for effective lake management.
Uncertainties and Limitations
The chloride mass balance model, though effective, is subject to uncertainties stemming from inherent variability in hydrological inputs and potential inaccuracies in tracer concentration data. External influences, such as seasonal shifts, land use changes, and transient groundwater flow dynamics, can further introduce deviations in the model’s estimates. These factors stress the need for cautious interpretation and the importance of validating the model with alternative tracers, such as radon (222Rn), or supplementing it with additional monitoring data. Radon, with its distinct properties, is particularly advantageous in differentiating groundwater sources and can provide a more robust assessment of groundwater-lake interactions. Additionally, the model’s reliance on steady-state conditions and consistent recharge-discharge pathways may overlook episodic fluctuations, potentially affecting the accuracy of long-term volume predictions. The predictive modeling approach used in the study recognizes uncertainties in parameter estimates and input variations, yet it maintains robustness and reliability, thereby enhancing confidence in the findings and their applicability to effective lake management strategies.
Implications for Sustainable Water Management and Future Directions
The results of this study underline the importance of sustainable water management practices tailored to the specific hydrological context of tropical environments. The integration of chloride mass balance and sensitivity analysis provides a comprehensive framework for understanding water dynamics, while the water balance model draws attention to the potential risks associated with current water use practices. Policymakers must prioritize groundwater recharge initiatives and adopt adaptive management strategies to enhance resilience against climatic fluctuations.
The identification of localized groundwater recharge and discharge processes in the northern (NE and NW) region of VL emphasizes the need for in-depth investigations with increased data granularity. To refine this understanding, future research should consider expanding the sampling regimen and adopting a multi-tracer approach. The inclusion of radon (222Rn) as an adjunct tracer is recommended for its potential to provide a more detailed examination of GW-SW interactions. This rigorous approach holds promise for elucidating the complexities of the hydrogeological network and enhancing the comprehensiveness of conceptual models. Such scientific rigor will contribute to the advancement of sustainable water resource management strategies tailored to the specific dynamics of Vellayani Lake.
This adaptable methodology is particularly well-suited for tropical lakes due to the complex hydrological dynamics typical of such environments, where groundwater-surface water interactions play a crucial role in maintaining water balance, enabling rapid and effective assessments. By addressing the role of groundwater in sustaining lake levels, the findings promote integrated water resource management strategies, ensuring the ecological and hydrological stability of tropical lake systems. The integrated approach of isotope analysis, chloride mass balance and ML offers a robust framework for studying GW-SW interactions in data-scarce tropical regions. ML methods like RF and K-means clustering proved highly accurate in identifying groundwater discharge zones, reducing reliance on extensive field campaigns.
Conclusion
Groundwater-surface water (GW-SW) interactions of a tropical lake, Vellayani Lake (VL), Kerala, India, were delineated using environmental tracers — stable water isotopes (s18O, sD) and chloride. The isotopic analysis indicated a predominant influence of precipitation on groundwater and a substantial influence of evaporation on lake water. The northern part of the lake emerged as a critical “hotspot”, indicating lacustrine groundwater discharge (LGD), necessitating targeted groundwater resource management interventions for sustainable lake maintenance, particularly during dry periods. The incorporation of machine learning techniques, including K-means clustering and Random Forest (RF) algorithms, further refined the classification of Lacustrine Groundwater Discharge (LGD) and non-Lacustrine Groundwater Discharge (non-LGD) sites.
The chloride-based mass balance analysis corroborated the significance of LGD as an essential input for the lake, particularly in the absence of riverine inputs, while also revealing substantial lake-to-groundwater recharge in the southern region. Furthermore, the validation metrics of the chloride mass balance model confirmed its effectiveness in accurately estimating GW-SW interactions.
The integration of predictive modeling with the One-At-a-Time (OAT) approach employing Sensitivity Indices elucidated precipitation and LGD as the major factors affecting lake volume dynamics. While not a conventional sensitivity analysis, this framework provides a structured examination of parameter-specific variations, offering critical insights into which factors most influence lake volume dynamics — essential for informed resource management and lake sustainability planning.
This study emphasizes the essential role of astute nearshore groundwater recharge and management in maintaining the hydrological balance of lakes in LGD-dominated regions in the northern part of the lake. The outcomes of this study are anticipated to catalyze more profound research endeavours, enhancing the broader understanding of GW-SW interactions in tropical lake ecosystems, particularly in data-scarce regions.
Acknowledgement
The authors gratefully acknowledge the University of Kerala, India, for providing the necessary facilities and resources to support this research.
Funding Sources
University of Kerala, India (Grant No. No.Ac E VII/3505/2024/UOK) for conducting this work.
Conflict of Interest
The author(s)do not have any conflict of interest.
Data Availability Statement
The manuscript includes all datasets generated or analyzed during this research study. Additional data will be made available upon request.
Ethics Statement
This research did not involve human participants, animal subjects, or any material that requires ethical approval.
Informed Consent Statement
This study did not involve human participants, and therefore, informed consent was not required.
Author Contributions
Sukanya S: Visualization, Methodology, Funding Acquisition, Writing- Original Draft.
Sabu Joseph: Conceptualization, Supervision, Project Administration, Writing- Original Draft, Review and Editing.
Saeid Eslamian: Analysis, Methodology, Writing- Review and Editing.
References
- Busker T, de Roo A, Gelati E, Schwatke C, Adamovic M, Bisselink B, Pekel JF, Cottam AA global lake and reservoir volume analysis using a surface water dataset and satellite altimetry. Hydrol Earth Syst Sci 2019;23(2):669-690.
CrossRef - Zhou W, Wang L, Li D, Leung LR. Spatial pattern of lake evaporation increases under global warming linked to regional hydroclimate change. Commun Earth Environ 2021;2(1):255.
CrossRef - Pham-Duc B, Sylvestre F, Papa F, Frappart F, Bouchez C, Crétaux JF. The Lake Chad hydrology under current climate change. Sci Rep 2020;10(1):1-10.
CrossRef - Wang S, He Y, Hu S, Ji F, Wang B, Guan X, Piccolroaz S. Enhanced warming in global dryland lakes and its drivers. Remote Sens 2021;14(1):86.
CrossRef - Papa F, Crétaux JF, Grippa M, Robert E, Trigg M, Tshimanga RM, Kitambo B, Paris A, Carr A, Fleischmann A. S, de Fleury M. Water resources in Africa under global change: monitoring surface waters from space. Surv Geophys 2023;44(1):43-93.
CrossRef - Lin Y, Gao C, Li X, Zhang T, Yu J, Rong Y, Li L, Zhou X, Cai J, Sneeuw N. Attribution Analysis of Climate Change and Human Activities to Water Volume Variation in Large Lakes. Int Arch Photogramm Remote Sens Spatial Inf Sci 2023;48:443-450.
CrossRef - Rahmani J, Danesh-Yazdi M. Quantifying the impacts of agricultural alteration and climate change on the water cycle dynamics in a headwater catchment of Lake Urmia Basin. Agric Water Manag 2022;270:107749.
CrossRef - Wang S, Xu C, Zhang W, Chen H, Zhang B. Human-Induced water loss from closed inland Lakes: Hydrological simulations in China’s Daihai lake. J Hydrol 2022;607:127552.
CrossRef - Ho L, Alonso A, Forio M. A. E, Vanclooster M, Goethals PL. Water research in support of the Sustainable Development Goal 6: A case study in Belgium. J Clean Prod 2020;277:124082.
CrossRef - Janssen A. B, Hilt S, Kosten S, de Klein J. J, Paerl HW, Van de Waal DB. Shifting states, shifting services: Linking regime shifts to changes in ecosystem services of shallow lakes. Freshwater Biol 2021;66(1):1-12.
CrossRef - Herrera C, Godfrey L, Urrutia J, Custodio E, Jordan T, Jódar J, Delgado K, Barrenechea F. Recharge and residence times of groundwater in hyper arid areas: The confined aquifer of Calama, Loa River Basin, Atacama Desert, Chile. Sci Total Environ 2021;752:141847.
CrossRef - Sabale R, Venkatesh B, Jose M. Sustainable water resource management through conjunctive use of groundwater and surface water: A review. Innov Infrastruct Solut 2023;8(1):17.
CrossRef - El Ouali A, Roubil A, Lahrach A, El Hmaidi A, El Ouali A, Ousmana H, Bouchaou L. Assessments of groundwater recharge process and residence time using hydrochemical and isotopic tracers under arid climate: Insights from Errachidia basin (Central-East Morocco). Groundwater Sustain Dev 2024;101145.
CrossRef - CWC (Central Water Commission). Reassessment of Water Availability in Basins using Spatial Inputs. Basin Planning & Management Organisation, New Delhi, India, 2017;100.
- Gupta R, Sharma PK. A review of groundwater-surface water interaction studies in India. J Hydrol 2023;129592.
CrossRef - Tigabu TB, Wagner PD, Hörmann G, Fohrer N. Modeling the spatio-temporal flow dynamics of groundwater-surface water interactions of the Lake Tana Basin, Upper Blue Nile, Ethiopia. Hydrol Res 2020;51(6):1537-1559.
CrossRef - Rodríguez-Rodríguez M, Aguilera H, Guardiola-Albert C, Fernández-Ayuso A. Climate influence vs. local drivers in surface water-groundwater interactions in eight ponds of Doñana National Park (southern Spain). Wetlands 2021;41(2):25.
CrossRef - Geris J, Comte JC, Franchi F, Petros AK, Tirivarombo S, Selepeng AT, Villholth KG. Surface water-groundwater interactions and local land use control water quality impacts of extreme rainfall and flooding in a vulnerable semi-arid region of Sub-Saharan Africa. J Hydrol 2022;609:127834.
CrossRef - Tigabu TB, Wagner PD, Hörmann G, Fohrer N. Sustainable Water Resources in Rural Areas: Impact of Land Use and Climate Change on Surface Water Groundwater Interactions at Lake Tana, Ethiopia. In: Sustainability of Water Resources: Impacts and Management. Cham, Germany: Springer International Publishing, 2022;375-399.
CrossRef - Duque C, Nilsson B, Engesgaard P. Groundwater–surface water interaction in Denmark. Wiley Interdiscip Rev Water 2023;10(5):e1664.
CrossRef - Sun X, Du Y, Deng Y, Fan H, Ma T. Contrasting lacustrine groundwater discharge and associated nutrient loads in different geological conditions. Hydrol Earth Syst Sci Discuss 2021;1-29.
CrossRef - Shi X, Luo X, Jiao JJ, Zuo J. Dominance of evaporation on lacustrine groundwater discharge to regulate lake nutrient state and algal blooms. Water Res 2022;219:118620.
CrossRef - Gu X, Xue M, Ye C, Jiao Y, Han Y, Wang W, Zhang X, Frape SK, Liu B. Evaluating heterogeneous lacustrine groundwater discharge of Hulun Lake using radium isotopes in Inner Mongolia, China. J Hydrol Reg Stud 2024;51:101650.
CrossRef - Yang J, Yu Z, Yi P, Frape SK, Gong M, Zhang Y. Evaluation of surface water and groundwater interactions in the upstream of Kui river and Yunlong lake, Xuzhou, China. J Hydrol 2020;583:124549.
CrossRef - Paíz R, Low-Calle JF, Molina-Estrada AG, Gil-Villalba S, de Melo MTC. Combining spectral analysis and geochemical tracers to investigate surface water–groundwater interactions: A case study in an intensive agricultural setting (southern Guatemala). Sci Total Environ 2023;899:165578.
CrossRef - Ntona MM, Busico G, Mastrocicco M, Kazakis N. Modeling groundwater and surface water interaction: An overview of current status and future challenges. Sci Total Environ 2022;846:157355.
CrossRef - Sukanya S, Noble J, Joseph S. Application of radon (222Rn) as an environmental tracer in hydrogeological and geological investigations: an overview. Chemosphere 2022;303:135141.
CrossRef - Jeelani G, Lone SA, Nisa AU, Deshpande RD, Padhya V. Use of stable water isotopes to identify and estimate the sources of groundwater recharge in an alluvial aquifer of Upper Jhelum Basin (UJB), western Himalayas. Hydrol Sci J 2021;66(16):2330-2339.
CrossRef - Liao F, Wang G, Yi L, Shi Z, Cheng G, Kong Q, Mu W, Guo L, Cheng K, Dong N, Liu C. Identifying locations and sources of groundwater discharge into Poyang Lake (eastern China) using radium and stable isotopes (deuterium and oxygen-18). Sci Total Environ 2020;740:140163.
CrossRef - Ren X, Yu R, Wang R, Kang J, Li X, Zhang P, Liu T. Tracing spatial patterns of lacustrine groundwater discharge in a closed inland lake using stable isotopes. J Environ Manag 2024;354:120305.
CrossRef - Sukanya S, Joseph S. Climate change impacts on water resources: An overview. In: Visualization Techniques for Climate Change with Machine Learning and Artificial Intelligence. 2023;55-76.
CrossRef - Li P, Zhang Y, Yang N, Jing L, Yu P. Major ion chemistry and quality assessment of groundwater in and around a mountainous tourist town of China. Exp Health 2016;8:239-252.
CrossRef - Oyarzún R, Barrera F, Salazar P, Maturana H, Oyarzún J, Aguirre E, Alvarez P, Jourde H, Kretschmer N. Multi-method assessment of connectivity between surface water and shallow groundwater: the case of Limarí River basin, north-central Chile. Hydrogeol J 2014;22(8):1857.
CrossRef - Iwanyshyn M, Ryan MC, Chu A. Separation of physical loading from photosynthesis/respiration processes in rivers by mass balance. Sci Total Environ 2008;390(1):205-214.
CrossRef - Baker J, Grasby SE, Ryan MC. Groundwater contribution keeps trophic status low in Sylvan Lake, Alberta, Canada. Can Water Resour J 2018;43(3):366-381.
CrossRef - Banerji US, Shaji J, Arulbalaji P, Maya K, Mohan SV, Dabhi AJ, Shivam A, Bhushan R, Padmalal D. Mid-late Holocene evolutionary history and climate reconstruction of Vellayani lake, south India. Quat Int 2021;599:72-94.
CrossRef - Veena MP, Achyuthan H, Eastoe C, Farooqui A. Human impact on low-land Vellayani Lake, south India: A record since 3000 yrs BP. Anthropocene 2014;8:83-91.
CrossRef - RStudio Team. RStudio: integrated development for R. RStudio Inc, Boston, MA, USA, 2023. Available at: http://www.rstudio.com/.
- Bruce LC, Frassl MA, Arhonditsis GB, Gal G, Hamilton DP, Hanson PC, Hetherington AL, Melack JM, Read JS, Rinke K, Rigosi A. A multi-lake comparative analysis of the General Lake Model (GLM): Stress-testing across a global observatory network. Environ Model Softw 2018;102:274-291.
CrossRef - Mahenthiran S, Ganesan M, Hameed AS. Environmental Isotopes of Water as Potential Tool to Distinguish the Sources of Groundwater Recharge in a Semi-arid Region: A Case Study Around Veeranam Tank, Tamil Nadu, India. J Inst Eng India Ser A 2023;104(4):831-839.
CrossRef - Das P, Mukherjee A, Hussain SA, Jamal MS, Das K, Shaw A, Layek MK, Sengupta P. Stable isotope dynamics of groundwater interactions with Ganges river. Hydrol Process 2021;35(1):e14002.
CrossRef - Qi S, Feng Q, Shu H, Liu W, Zhu M, Zhang C, Yang L, Yin Z. Redistribution effect of irrigation on shallow groundwater recharge source contributions in an arid agricultural region. Sci Total Environ 2023;865:161106.
CrossRef - Dar T, Rai N, Bhat MA, Kumar S, Jahan A. Surface water isoscape modeling in the Himalayan region: An alternative for moisture source investigation, hydrograph separation, and paleolatitude estimation. Water Resour Res 2023;59(6):e2022WR033572.
CrossRef - Loh YSA, Fynn OF, Manu E, Afrifa GY, Addai MO, Akurugu BA, Yidana SM. Groundwater-surface water interactions: application of hydrochemical and stable isotope tracers to the lake Bosumtwi area in Ghana. Environ Earth Sci 2022;81(22):518.
CrossRef - O'Connell DW, Rocha C, Daly E, Carrey R, Marchesi M, Caschetto M, Ansems N, Wilson J, Hickey C, Gill LW. Characterization of seasonal groundwater origin and evolution processes in a geologically heterogeneous catchment using geophysical, isotopic and hydro?chemical techniques (Lough Gur, Ireland). Hydrol Process 2022;36(10):e14706.
CrossRef - Dansgaard W. Stable isotopes in precipitation. Tellus 1964;16(4):436-468.
CrossRef - Yuan F, Sheng Y, Yao T, Fan C, Li J, Zhao H, Lei Y. Evaporative enrichment of oxygen-18 and deuterium in lake waters on the Tibetan Plateau. J Paleolimnol 2011;46:291-307.
CrossRef - Wu H, Huang Q, Fu C, Song F, Liu J, Li J. Stable isotope signatures of river and lake water from Poyang Lake, China: Implications for river–lake interactions. J Hydrol 2021;592:125619.
CrossRef - Zolkos S, MacDonald E, Hung JK, Schade JD, Ludwig S, Mann PJ, Treharne R, Natali S. Physiographic controls and wildfire effects on aquatic biogeochemistry in Tundra of the Yukon?Kuskokwim Delta, Alaska. J Geophys Res Biogeosciences 2022;127(8):e2022JG006891.
CrossRef - Zhan L, Chen J, Zhang S, Huang D, Li L. Relationship between Dongting Lake and surrounding rivers under the operation of the Three Gorges Reservoir, China. Isotopes Environ Health Stud 2015;51(2):255-270.
CrossRef - Vystavna Y, Schmidt SI, Kopá?ek J, Hejzlar J, Holko L, Matiatos I, Wassenaar LI, Persoiu A, Badaluta CA, Huneau F. Small-scale chemical and isotopic variability of hydrological pathways in a mountain lake catchment. J Hydrol 2020;585:124834.
CrossRef - Qiu X, Peng W, Sun L, Chen K, Zhao X. Use of hydrogen and oxygen isotopes to understand evaporation from enclosed waterbodies. J Environ Eng Landscape Manag 2022;30(1):220-225.
CrossRef - Xiang Q, Liu G, Meng Y, Chen K, Xia C. Temporal trends of deuterium excess in global precipitation and their environmental controls under a changing climate. J Radioanal Nucl Chem 2022;331(9):3633-3649.
CrossRef - Heydarizad M, Minaei M, Ichiyanagi K, Sorí R. The effects of local and regional parameters on the ?18O and ?2H values of precipitation and surface water resources in the Middle East. J Hydrol 2021;600:126485.
CrossRef - Mohamed MM, Parimalarenganayaki S, Khan Q, Murad A. Review on the use of environmental isotopes for groundwater recharge and evaporation studies in the GCC countries. Groundwater Sustain Dev 2021;12:100546.
CrossRef - Natali S, Doveri M, Giannecchini R, Baneschi I, Zanchetta G. Is the deuterium excess in precipitation a reliable tracer of moisture sources and water resources fate in the western Mediterranean? New insights from Apuan Alps (Italy). J Hydrol 2022;614:128497.
CrossRef - Rejith RG, Sundararajan M, Gowtham B, Balasubramanian A, Lawrence JF. Characterization of weathering profile and quality of groundwater over hard rock terrain. Arab J Geosci 2021;14:1-12.
CrossRef - Meyer R, Engesgaard P, Sonnenborg TO. Origin and dynamics of saltwater intrusion in a regional aquifer: Combining 3?D saltwater modeling with geophysical and geochemical data. Water Resour Res 2019;55(3):1792-1813.
CrossRef - Wallace H, Ji T, Robinson CE. Hydrogeological controls on heterogeneous groundwater discharge to a large glacial lake. J Great Lakes Res 2020;46(3):476-485.
CrossRef - Rey N, Rosa E, Cloutier V, Lefebvre R. Using water stable isotopes for tracing surface and groundwater flow systems in the Barlow-Ojibway Clay Belt, Quebec, Canada. Can Water Resour J 2018;43(2):173-194.
CrossRef - Sukanya S, Noble J, Joseph S. Factors controlling the distribution of radon (222Rn) in groundwater of a tropical mountainous river basin in southwest India. Chemosphere 2021;263:128096.
CrossRef - Hsu S. M, Ke CC, Dong MC, Lin YT. Investigating fault zone hydraulic properties and groundwater potential in a fault-dominated aquifer system: A case study of the Dili fault in Central Taiwan. Eng Geol 2022;308:106805.
CrossRef - Hare DK, Boutt DF, Clement WP, Hatch CE, Davenport G, Hackman A. Hydrogeological controls on spatial patterns of groundwater discharge in peatlands. Hydrol Earth Syst Sci 2017;21(12):6031-6048.
CrossRef - Karan S, Kidmose J, Engesgaard P, Nilsson B, Frandsen M, Ommen DAO, Flindt MR, Andersen FØ, Pedersen O. Role of a groundwater–lake interface in controlling seepage of water and nitrate. J Hydrol 2014;517:791-802.
CrossRef - Graillot D, Paran F, Bornette G, Marmonier P, Piscart C, Cadilhac L. Coupling groundwater modeling and biological indicators for identifying river/aquifer exchanges. SpringerPlus 2014;3:1-14.
CrossRef - Périllon C, Pöschke F, Lewandowski J, Hupfer M, Hilt S. Stimulation of epiphyton growth by lacustrine groundwater discharge to an oligo-mesotrophic hard-water lake. Freshwater Sci 2017;36(3):555-570.
CrossRef - Périllon C, van de Weyer K, Päzolt J, Kasprzak P, Hilt S. Changes in submerged macrophyte colonization in shallow areas of an oligo-mesotrophic lake and the potential role of groundwater. Limnologica 2018;68:168-176.
CrossRef - Goni IB, Taylor RG, Favreau G, Shamsudduha M, Nazoumou Y, Ngounou Ngatcha B. Groundwater recharge from heavy rainfall in the southwestern Lake Chad Basin: evidence from isotopic observations. Hydrol Sci J 2021;66(8):1359-1371.
CrossRef - Ma Z, Wang W, Hou X, Wang J, Duan L, Wang Y, Zhao M, Li J, Jing J, Li L. Examining the change in groundwater flow patterns: A case study from the plain area of the Baiyangdian Lake Watershed, North China. J Hydrol 2023;625:130160.
CrossRef - Smith RL, Repert DA, Stoliker DL, Kent DB, Song B, LeBlanc DR, McCobb TD, Böhlke JK, Hyun SP, Moon HS. Seasonal and spatial variation in the location and reactivity of a nitrate?contaminated groundwater discharge zone in a lakebed. J Geophys Res Biogeosci 2019;124(7):2186-2207.
CrossRef - Woolway RI, Kraemer BM, Lenters JD, Merchant CJ, O’Reilly CM, Sharma S. Global lake responses to climate change. Nat Rev Earth Environ 2020;1(8):388-403.
CrossRef - Musie M, Momblanch A, Sen S. Exploring future global change-induced water imbalances in the Central Rift Valley Basin, Ethiopia. Clim Chang 2021;164(3):47.
CrossRef - Goharian E, Burian SJ, Bardsley T, Strong C. Incorporating potential severity into vulnerability assessment of water supply systems under climate change conditions. J Water Resour Plan Manag 2016;142(2):04015051.
CrossRef - Wang Z, Yang Y, Chen G, Wu J, Wu J. Variation of lake-river-aquifer interactions induced by human activity and climatic condition in Poyang Lake Basin, China. J Hydrol 2021;595:126058.
CrossRef